Vector Search
Nomic Atlas enables you to search your dataset semantically with vector search.
You can run neural search over embeddings generated by Nomic Embedding models or your own.
In this example, we create a dataset of 25,000 news articles with the default Nomic Text Embedding model and run various types of semantic search.
from nomic import atlas
import pandas
news_articles = pandas.read_csv('https://raw.githubusercontent.com/nomic-ai/maps/main/data/ag_news_25k.csv')
dataset = atlas.map_data(data=news_articles, indexed_field='text')
print(dataset)
Atlas Interface
Vector search is a readily-available functionality in the Atlas interface. Use vector search in Atlas to visualize semantic neighborhoods and create complex data selections.
Vector Search Tool
Open the vector search modal by clicking its selection icon or using the hotkey 'V'.
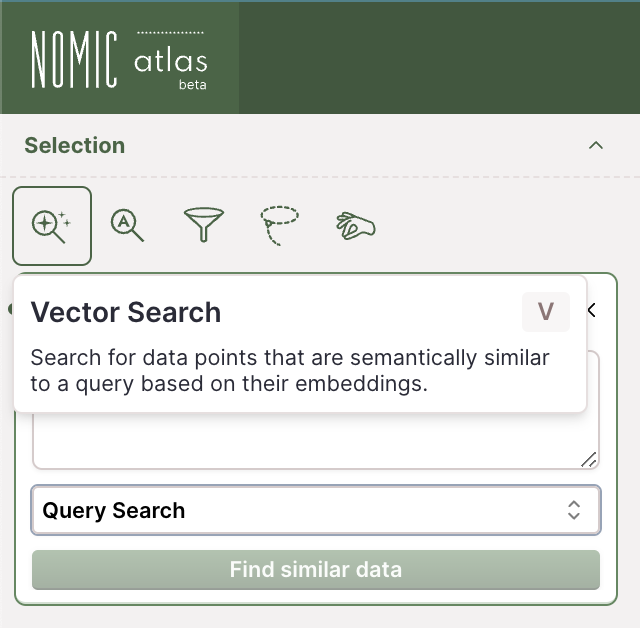
Vector search has three modes:
-
Query Search (Default): Find data points that best answer a question you have about your data (e.g. "What's happening with the stock market?")
-
Document Search: Find data points that are most similar to a text sample you provide. (e.g. "Stock market performance")
-
Embedding Search: Find data points that are close to a user-inputted embedding (e.g.
[0.42, 0.87, 0.013, ...]
). This is the only mode supported for Embedding datasets. The search will fail or provide incorrect results if your embedding does not have the same dimensionality as your dataset's embeddings or comes from a different embedding model/space.
After submitting a successful search, a slider will appear for you to adjust. The slider is over similarity values (i.e. dot products) where the larger the value, the more similar the data point is to the search vector. Drag the slider to the left to include data points that are less similar and right for more similar. The percentages displayed shows the percentile number of data points captured between the similarity cutoffs. The Atlas map selection will automatically update based on the slider range.
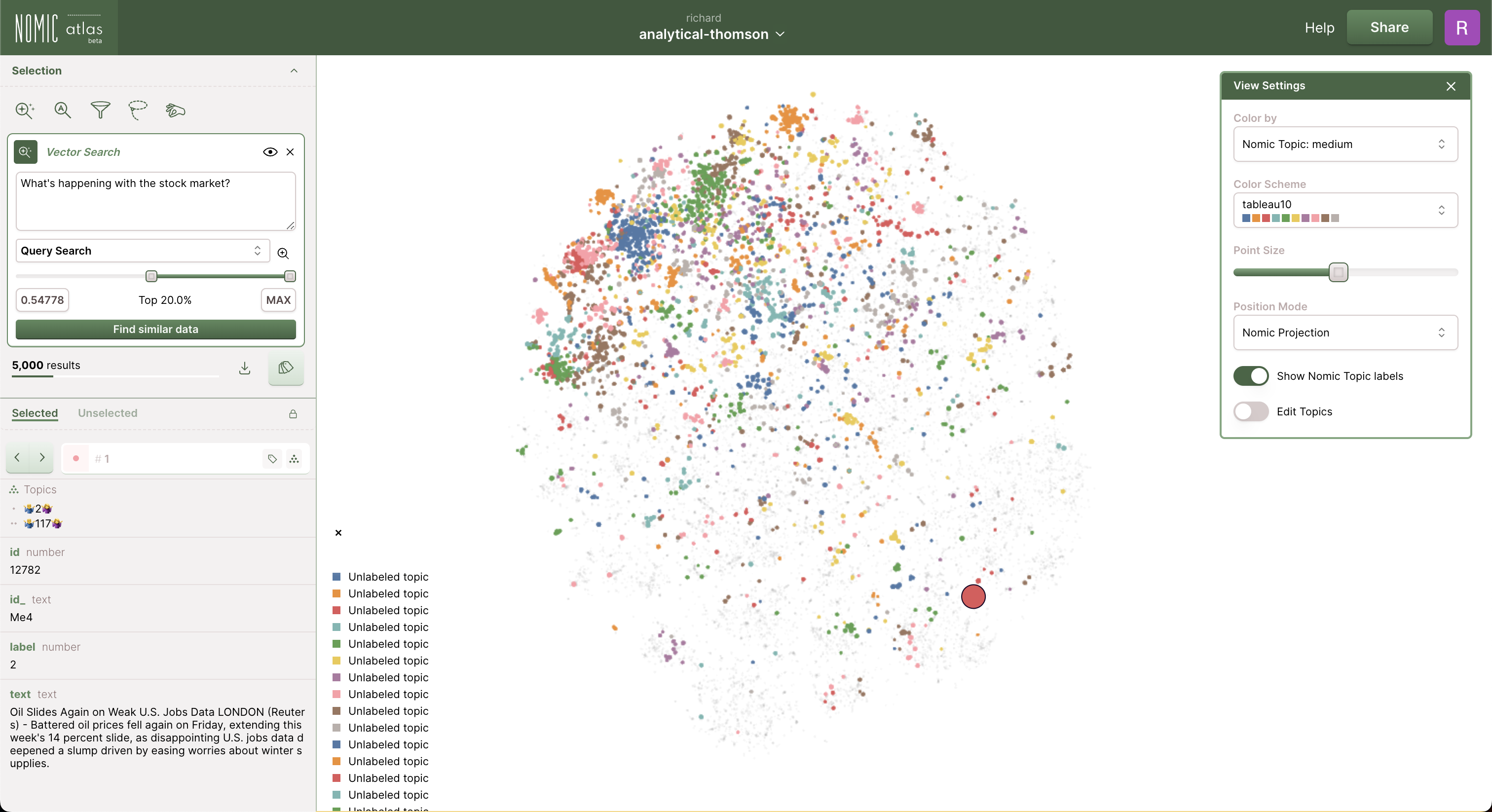
Vector Search as a Filter
Vector Search is part of the selection paradigm within Atlas. This means that out-of-the-box, you can combine your vector search filter with other Atlas tools to compose complex data selections.
Below, we join our vector search results with a exact term search and a visual lasso to find a subset of technology stock articles we are interested in.
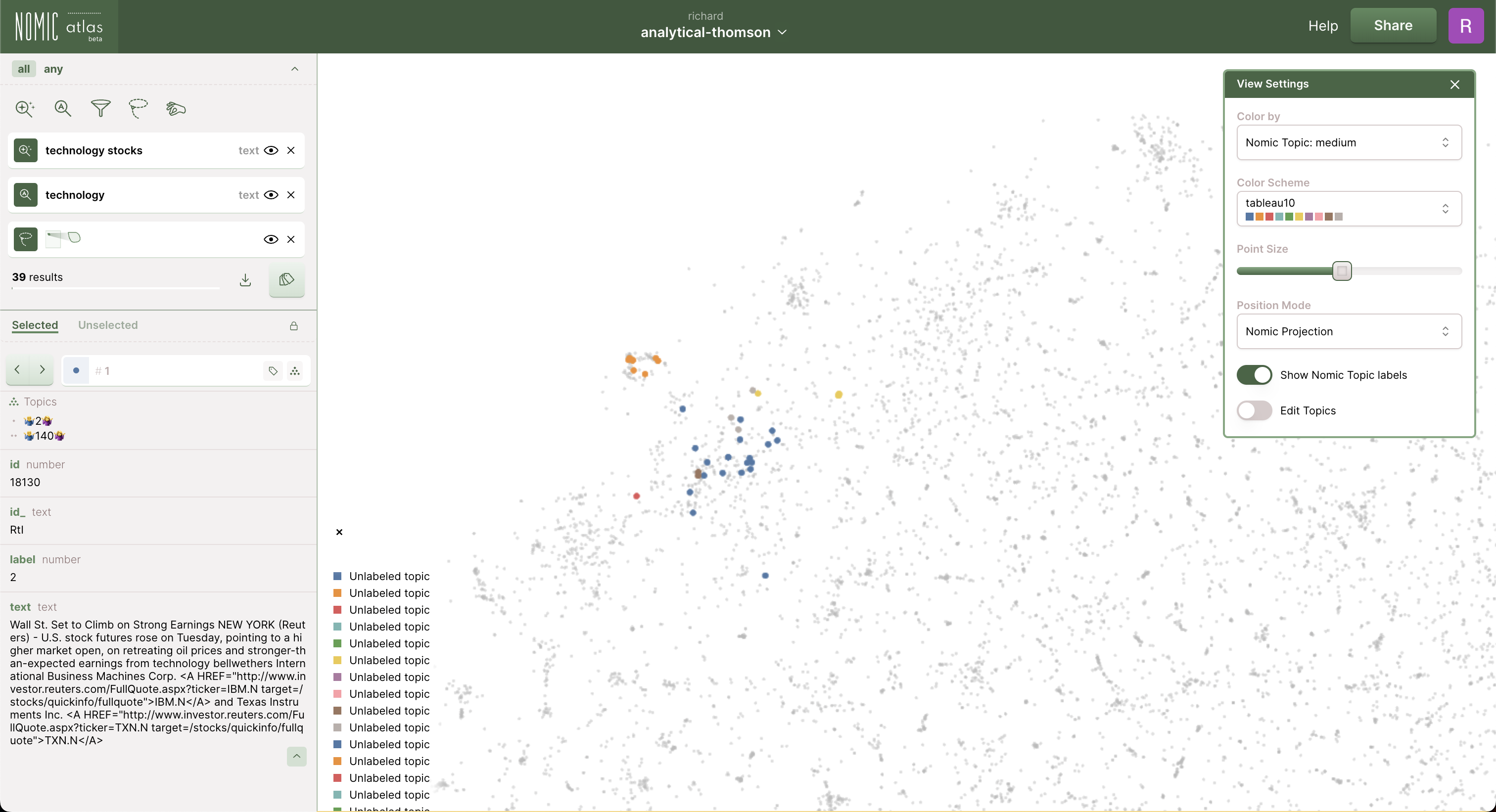
Nomic Multimodality
Nomic Text and Vision embedding models provide compatible, aligned embeddings (See details here). This means you can run text-to-image and image-to-text vector searches on your data (e.g. Find cat articles by providing a picture of a cat; find cat images that match the query "What animals are cute to cuddle with?").
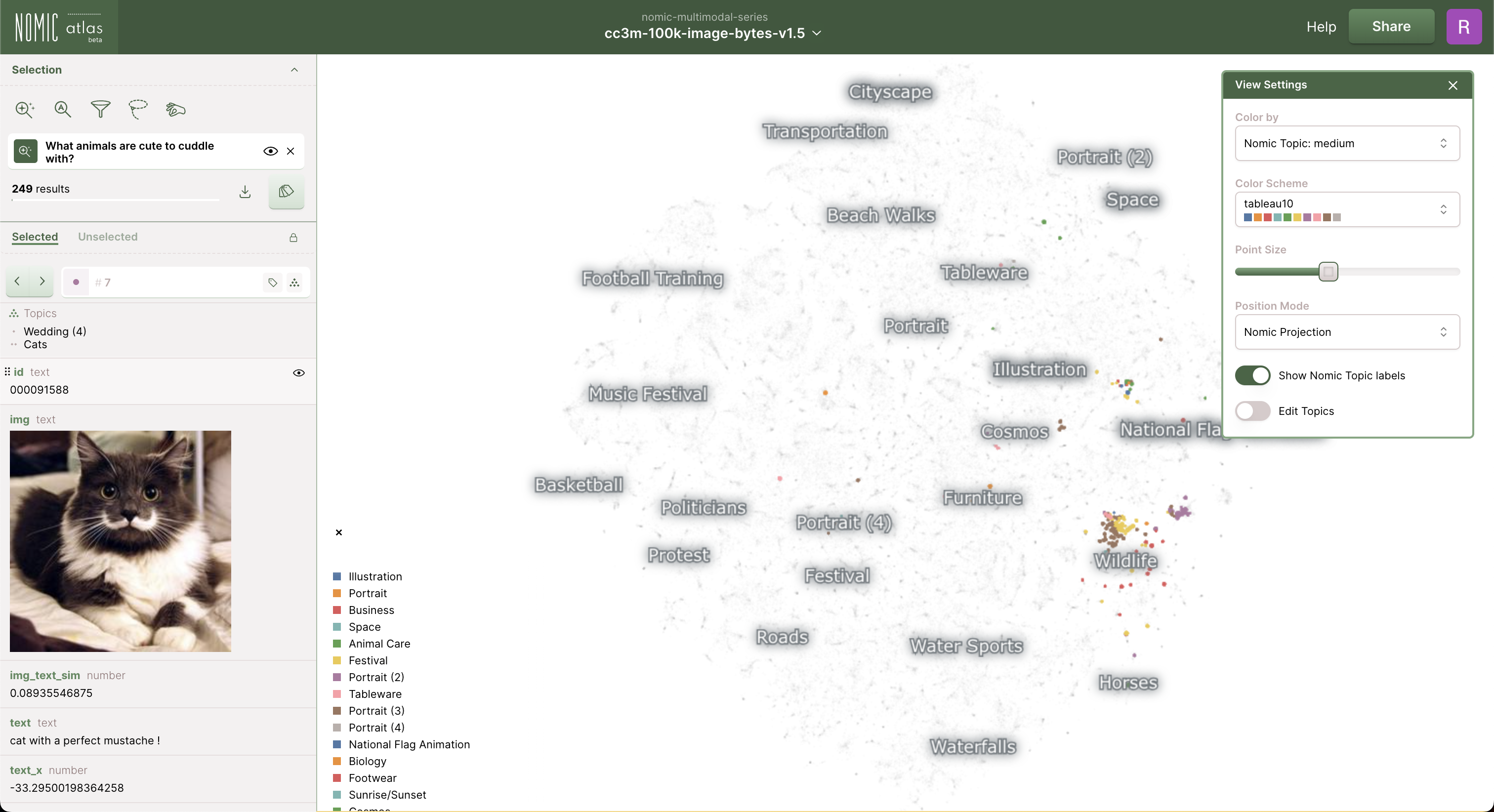
Python
Searching by datapoint
Running vector_search
on your dataset will return the IDs of the k-closest datapoints to a given query point.
- Python
- Output
# Load map and perform vector search
map = dataset.maps[0]
# Run vector search on your map for points with ID numbers 100, 111, 112
neighbors, distances = map.embeddings.vector_search(ids=[100,111,112], k=5)
# `neighbors` is a list (by ID) of neighborhoods of
# size k points for each of your vector search queries
[['100', '3302', '6992', '12687', '21208'], # Neighborhood of datum ID "100"
['111', '11077', '16377', '10822', '12853'], # Neighborhood of datum ID "111"
['112', '12172', '8418', '1109', '3798']] # Neighborhood of datum ID "112"
From IDs, print the values of the datapoints:
- Python
- Output
# Print the 5 most similar datapoints to the data point to your first query point (id #100)
# Your query: 'The US team is set for Spain for the Davis Cup final NEW YORK Andy Roddick and Mardy Fish will represent the United States in singles play at next month #39;s Davis Cup final against Spain.',
similar_datapoints = dataset.get_data(ids=neighbors[0])
for i, point in enumerate(similar_datapoints):
if i == 0:
print('Initial point:',point,'\n')
print('Nearest neighbors:')
else:
print(point)
Initial point: {'text': 'The US team is set for Spain for the Davis Cup final NEW YORK Andy Roddick and Mardy Fish will represent the United States in singles play at next month #39;s Davis Cup final against Spain.', 'label': 1, 'id': '100'}
Nearest neighbors:
{'text': "U.S. Gets Spain in Final The United States completes a 4-0 sweep over Belarus Sunday after Andy Roddick's win, and heads to Spain for the Davis Cup finals.", 'label': 1, 'id': '3302'}
{'text': 'US will battle host Spaniards in Seville Madrid, Spain (Sports Network) - The United States and Spain will play their 2004 Davis Cup final on a clay court at Olympic Stadium in Seville, from December 3-5.', 'label': 1, 'id': '6992'}
{'text': 'US hosts Croatia in next year #39;s Davis Cup 1st round Top-seeded Spain plays at Slovakia, while No. 2 United States hosts Croatia in the opening round of next year #39;s Davis Cup World Group.', 'label': 1, 'id': '12687'}
{'text': 'Spain vs US in Seville for Davis Cup title Spain will take on the United States for the Davis Cup champion in Southern Spain #39;s Seville City on December 3-5, China Radio International reported Wednesday.', 'label': 1, 'id': '21208'}
Searching by embedding
You may also vector search using a query vector instead of an ID. This function finds nearest neighbors based on your input vector.
- Python
- Output
import numpy as np
# Generates a random query vector
random_query_vector = np.random.rand(1, 768)
# Searches for k-nearest neighbors of random_query_vector
with dataset.wait_for_dataset_lock():
neighbors, distances = map.embeddings.vector_search(queries=random_query_vector, k=10)
print("Neighbor IDs:", neighbors)
data = dataset.get_data(ids=query_document_ids)
for datum, datum_neighbors in zip(data, neighbors):
neighbor_data = dataset.get_data(ids=datum_neighbors)
print(f"The ten nearest neighbors to the query point {datum} are {neighbor_data}")
Neighbor IDs: [['14628', '752', '17918', '17422', '1231', '745', '16454', '9881', '10312', '3698']]
The ten nearest neighbors to the query point {'text': 'First class to the moon London - British airline magnate Richard Branson announced a plan on Monday for the world #39;s first commercial space flights, saying quot;thousands quot; of fee-paying astronauts could be sent into orbit in the near future.', 'label': 3, 'id': '0'} are [{'text': "IBM Supercomputer Again Claims Record A \\$100 million supercomputer being built to analyze the nation's nuclear stockpile has again set an unofficial performance record - the second in just over a month.", 'label': 3, 'id': '14628'}, {'text': 'Big Blue Reveals Fastest Supercomputer Alive In a historic development in the computing world, IBM has succeeded in re-writing the rulebook for ultra-powerful computing by developing the worlds most powerful supercomputer- Blue Gene/L supercomputer, surpassing NECs Earth Simulator in Japan.', 'label': 3, 'id': '752'}, {'text': "Photos: IBM's Blue Gene/L supercomputer Sixteen racks of IBM's Blue Gene/L supercomputer can perform 70.7 trillion calculations per second.", 'label': 3, 'id': '17918'}, {'text': 'IBM Puts Blue Genes on the Shelf IBM said in a press release that it is working with many national lab and universities to develop Blue Gene supercomputer applications in areas such as life sciences, hydrodynamics, quantum ', 'label': 3, 'id': '17422'}, {'text': 'IBM to commercialize Blue Gene supercomputer (InfoWorld) InfoWorld - Fresh from setting a record for performance among supercomputers just a few days ago, IBM on Monday announced it is making a commercial version of its Blue Gene system available to be aimed at businesses and scientific researchers.', 'label': 3, 'id': '1231'}, {'text': 'IBM Adds Downtime Safety To WebSphere IBM has added a new feature to WebSphere Application Server designed to help safeguard Internet business applications against outages.', 'label': 3, 'id': '745'}, {'text': 'UK Official Confirms Minister Blunkett Resigned LONDON (Reuters) - Senior British government minister David Blunkett, a trusted ally of Prime Minister Tony Blair, resigned on Wednesday, a government official confirmed.', 'label': 0, 'id': '16454'}, {'text': 'SGI supercomputer: Two records in one day The public record has been eclipsed by a faster result yet to be announced, CNET News.com has learned.', 'label': 3, 'id': '9881'}, {'text': 'IBM pushes out new Stinger database SEPTEMBER 09, 2004 (COMPUTERWORLD) - IBM said today that it #39;s about to ship the next generation of its DB2 Universal Database, which it said offers self-tuning capabilities that will result in less management overhead even as databases grow bigger and ', 'label': 3, 'id': '10312'}, {'text': "IBM discloses details of chip (SiliconValley.com) SiliconValley.com - When IBM gave a sneak peek at its new Cell microprocessor this week, it was short on specifics about what it calls a supercomputer on a chip. But details are leaking out thanks to a recent patent awarded to IBM and Big Blue's own disclosures for an upcoming conference.", 'label': 3, 'id': '3698'}]
Retrieving search distances
- Python
- Output
# Load map and perform vector search
map = dataset.maps[0]
with dataset.wait_for_dataset_lock():
neighbors, distances = map.embeddings.vector_search(ids=[100,111,112], k=5)
print(distances)
# `distances` is a list of Euclidean distance values of size k points
# for each of your vector search queries
[[0.0,
0.7122321724891663,
0.7326422333717346,
0.7438449859619141,
0.744426429271698],
[0.0,
0.9628511071205139,
0.9816097021102905,
0.9883016347885132,
1.0143767595291138],
[0.0,
0.3459359109401703,
0.3928901255130768,
0.4260229170322418,
0.4471500515937805]]